How can AI improve drug optimization with symptom scoring
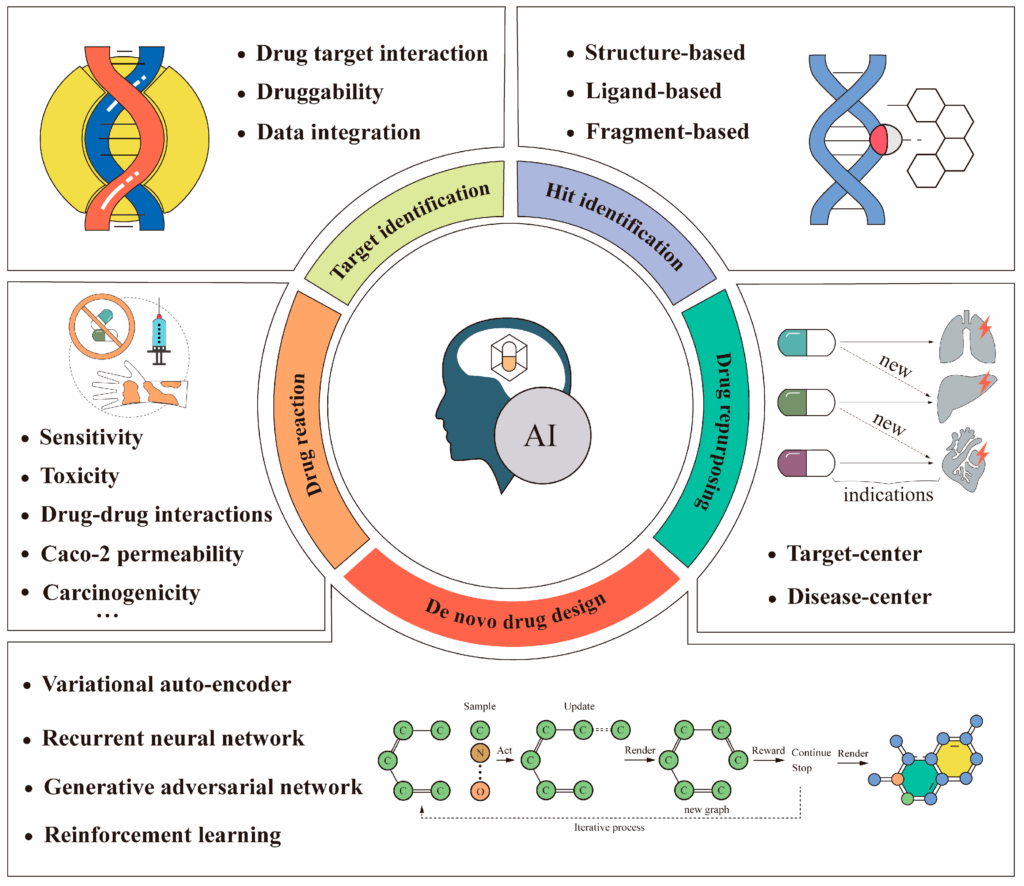
Intro
Increasing medication optimization using symptom rating presents several modern difficulties for the pharmaceutical and healthcare industries. The complex interplay between clinical data and human biology makes it difficult to link effective pharmacological treatments to particular symptoms. Currently, there is a need for huge high-quality labeled datasets and robust symptom representations to leverage artificial intelligence (AI) and machine learning for symptom-based drug discovery. The “black-box” nature of deep learning models makes it more challenging to comprehend and rely on predictions, underscoring the necessity of transparent and intelligible artificial intelligence systems.
The digitalization of data in the pharmaceutical industry has grown significantly, but it also makes it harder to learn and use that information to address challenging clinical situations. Large amounts of data are handled with improved automation thanks to artificial intelligence (AI), a technology-based system that mimics human intelligence. To read and learn from input data and make independent judgments for particular goals, AI requires systems and software. Automation that is guided by AI is advancing quickly, which could alter work culture.
Table of Contents
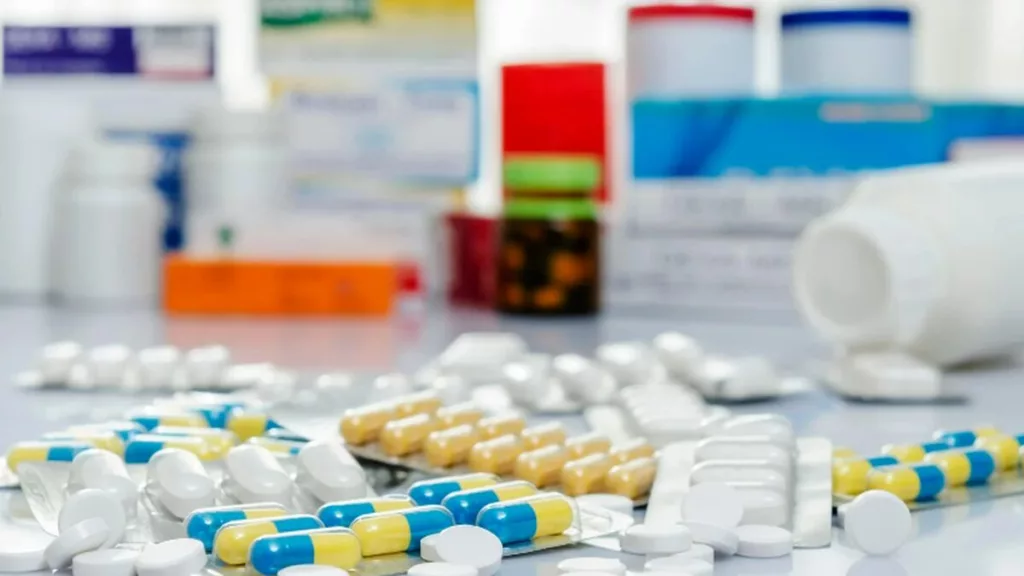
AI encompasses a variety of approach disciplines, including knowledge representation, problem-solving, and reasoning. An essential AI paradigm is machine learning (ML), which uses algorithms to find patterns in data. Artificial neural networks (ANNs), which are interconnected computational components with perceptons comparable to human biological neurons, are used in the deep learning (DL) discipline of machine learning. Convolutional neural networks (CNNs), recurrent neural networks (RNNs), and multilayer perceptron (MLP) networks are three types of ANNs that each have supervised and unsupervised training methods. While RNNs are closed-loop networks that can memorize and store information, MLP networks are utilized for pattern recognition, optimization assistance, process identification, and controls. In signal processing, biological system modeling, and image and video processing, CNNs are dynamic systems having local connections.
For example, AI-based solutions, like the IBM Watson supercomputer, evaluate patient medical data and compare it to a massive database to provide therapy recommendations for cancer. By detecting breast cancer in just 60 seconds, this device also helps in the early detection of diseases.
AI accelerating drug discovery
AI encompasses a variety of approach disciplines, including knowledge representation, problem-solving, and reasoning. An essential AI paradigm is machine learning (ML), which uses algorithms to find patterns in data. Artificial neural networks (ANNs), which are interconnected computational components with perceptons comparable to human biological neurons, are used in the deep learning (DL) discipline of machine learning. Convolutional neural networks (CNNs), recurrent neural networks (RNNs), and multilayer perceptron (MLP) networks are three types of ANNs that each have supervised and unsupervised training methods. While RNNs are closed-loop networks that can memorize and store information, MLP networks are utilized for pattern recognition, optimization assistance, process identification, and controls. In signal processing, biological system modeling, and image and video processing, CNNs are dynamic systems having local connections. For example, AI-based solutions, like the IBM Watson supercomputer, evaluate patient medical data and compare it to a massive database to provide therapy recommendations for cancer. By detecting breast cancer in just 60 seconds, this device also helps in the early detection of diseases.
Impacting the workflows of pathologist
Clinical oncology uses artificial intelligence (AI) for various purposes, including digital pathology, biomarker creation, therapy, etc. While biomarker research has been applied to immune checkpoint inhibition and the prediction of ovarian cancer, AI-based pathology has shown promise in assisting clinician-driven risk prediction and diagnosis. AI has also shown promising improvements in drug development, including the identification of targets and the creation of innovative treatments for a variety of disease indications. Recognizing AI’s importance across the entire therapeutic spectrum, from discovery to development, is essential to establish. AI as a platform for improving cancer treatment. Other growth areas, like data infrastructures, data kinds, and patient matching, may also use AI.
AI has dramatically improved conventional cancer diagnosis and prognosis by enabling the validation of digital pathology as a prognostic platform and the development of digital biomarkers
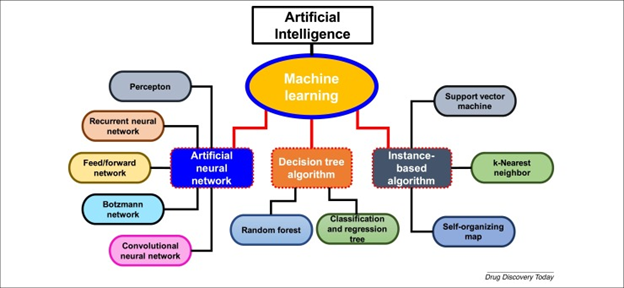
DL in cancer pathology for the development of digital biomarkers
Finding molecular biomarkers for personalized cancer treatment is a challenge for artificial intelligence in oncology. Advanced tumor cell analysis using deep learning (DL) techniques is being investigated. These techniques include inference of molecular characteristics, mutation prediction, survival prediction, and therapeutic response prediction. Prescreening patients using DL-based genotyping has the potential to take the role of conclusive testing techniques. Large-scale clinical validation to verify potential biomarker impact is a challenge.
Using imaging of the tumor vascular system, develop biomarkers.
A tumor vasculature-based biomarker was created in a study to forecast patient response and outcome prognosis. NSCLC patients on immune checkpoint inhibitor therapy were examined using quantitative vascular tortuosity (QVT). However, additional prospective clinical validation is needed because more tissue is required for Analysis. This is a positive breakthrough for the creation of digital biomarkers. A study investigated the relationship between collagen fiber digital pathology and breast cancer outcomes. The extracellular matrix’s most prevalent protein, collagen, has been examined using laser scanning microscopy or second harmonic generation (SHG)-based microscopy. These techniques are only sometimes applied in clinical practice, nevertheless. The study investigated the relationship between collagen fiber organization and outcomes for disease-free survival using conventional hematoxylin and eosin staining.
DL accuracy issues and improving digital pathology reliability
The creation of clinical biomarkers, gene expression patterns, survival rates, and harmful mutations may be made possible by the potential for digital histopathology (DL) to uncover minor but distinct features in tumor subtypes. Over 10,000 digital slides of 24 different types of tumors are included in The Cancer Genome Atlas (TCGA), a sizable digital histology collection that includes pertinent clinical, genomic, and radiomic information. However, altering histological specimens for digital imaging might result in differences in the produced images, creating site-specific digital histology signatures. This may result in biased feature prediction accuracy in cancer research. Several measures have been taken to lessen prejudice and increase reliability in identifying patient specifics to achieve equitable DL application and accurate forecasts. The ability of digital histopathology (DL) to identify minute but distinctive features in cancer subtypes may enable the development of clinical biomarkers, gene expression patterns, survival rates, and dangerous mutations. The Cancer Genome Atlas (TCGA), a substantial digital histology collection with pertinent clinical, genomic, and radiomic data, has over 10,000 digitized slides of 24 types of cancers. However, modifying histological samples for digital imaging may lead to variations in the generated images, creating site-specific digital histology signatures. In cancer research, this could lead to biased feature prediction accuracy. To ensure equitable DL application and precise projections, several steps have been taken to reduce prejudice and improve the reliability of detecting patient details.
Clinical cancer treatment has advanced using AI
The use of AI in drug discovery, development, and dosing is highlighted in this section’s discussion of integrating drug discovery and development in cancer treatment. It also outlines a framework for the most effective clinical cancer treatment, guided by AI-enhanced diagnostic and biomarker development capabilities.
- Utilizing AI to Create and Find New Therapies – A study shows the value of combining the AI-powered drug discovery systems PandaOmics and Chemistry42 with the AI-based protein structure prediction system AlphaFold. Within 30 days, this resulted in the discovery of a new hit chemical for treating hepatocellular carcinoma against a novel target.
- AI-Based Approach to Cancer Therapy Regimen Design- Developing the quadratic phenotype optimization platform (QPOP) addressed the low response rates of chemotherapy-resistant patients in clinical practice. Ex vivo testing of biopsied tumor samples is done after designing patient-specific medication combinations using laboratory experiments and optimization analysis. To rank drug combinations for specific patients and to allow for individualized drug-drug interactions, QPOP uses an orthogonal array composite design to decrease experimental data points from huge parameter spaces. A prospective investigation revealed that 67 of 75 patient samples used QPOP to produce complete responses, suggesting the possibility of further, extensive validation studies. Another study identified ex vivo medication efficacy without using population-scale data or genomic information by drug profiling patient samples using an image-based single-cell functional precision medicine platform.
Talking about Cancer Therapy Dosage for AI
To help cancer cells receive better treatment, evolutionary game theory is suggested as a model for medical therapies and cancer cell resistance tactics. This method overcomes the drawback of conventional treatment regimens, emphasizing short-term achievement but failing to account for tumor features over the long run. According to game theory, dynamic treatment regimens and methods for managing resistance are essential. The relationship between glucose membrane transporters and cancer cells’ proliferative and metastatic potential has also been studied. Studies using game theory have significantly enhanced clinical trials.
Use cases in oncology
Oncology AI-based models can enhance patient outcomes and maximize therapies. However, proving their efficacy and safety requires critical considerations, including data quality, accuracy, reliability, and ethical and legal ramifications. For trust and patient-centered care, open communication and transparency are essential. Protecting patients’ privacy and balancing advancement and security are crucial factors in incorporating AI.
The use case for I3LUNG
The Horizon Europe (Europe) call “Ensuring access to innovative, sustainable, and high-quality health care” provided funding for the European project I3LUNG. By offering predictive models, individualized decision-making platforms, and safe data access for research, the I3LUNG Project, sponsored by Horizon Europe, intends to incorporate AI into clinical oncology. The study combines retrospective, observational, and prospective validation to determine the value of big data and diverse data sources for individualized prediction in patients with advanced non-small-cell lung cancer treated with immunotherapy. The finished product intends to develop an integrated CDS platform and AI-assisted data storage for IO-based therapy in NSCLC.
Applications of AI-based oncology: Challenges and Opportunities
Clinical cancer diagnosis, treatment, and monitoring may benefit from using AI. However, issues such as clinical validation, scaling up integration into clinical workflows, and guaranteeing physician and patient confidence in AI-based suggestions remain resolved. Additional verification is required to create biomarkers and the predictive matching of patients to medicines. AI-driven medication discovery may not be sufficient to improve patient care, but developing a thorough workflow that guarantees effective compound management is essential. In contrast to conventional mechanism-of-action-based combination design, AI has redesigned pharmacological combinations, uncovering unanticipated drug interactions that boost therapeutic efficacy. However, there are still practical difficulties in implementing mechanism independence into standard drug development procedures via Clinical Development Systems (CDS).
Post hoc explainable AI (XAI) aims to increase the usability of ML and DL models by making them transparent, interpretable, accountable, and understandable. Oncology research employs XAI techniques like saliency maps and SHAP to explain DL model predictions. Future advancements in XAI in medicine will increase AI’s openness, responsibility, and reliability in healthcare.
Legal and Ethical Considerations & Potential Benefits of AI in Clinical Oncology
Several moral and legal issues regarding the use of AI in clinical research need to be addressed. Patient privacy preservation is a critical issue since enormous amounts of potentially sensitive patient data can be gathered. The susceptibility of data to cyberattacks is another factor. Therefore, it is crucial to have rigorous privacy regulations and safeguards against data theft. It will be necessary to consider and discuss additional issues, such as data ownership and patient access to their data.
AI can enhance evidence-based oncology care by empowering the clinical community to improve patient outcomes, therapy selection, and drug regimen selection. The discovery of biomarkers and images with AI assistance can improve predictive capabilities and save lives. Additional effort is required across various disciplines to expand these prospects and alter clinical oncology practice.
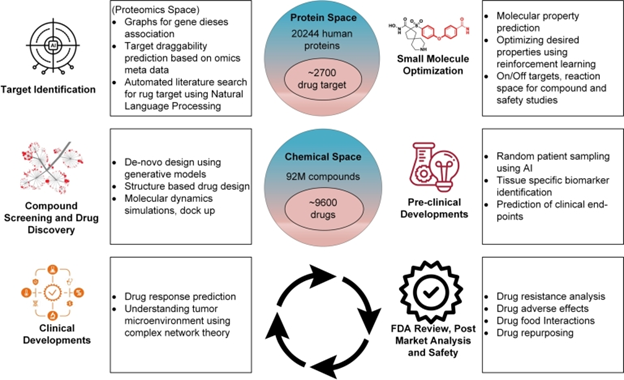
Topological examination of data
Topological data analysis (TDA) examines large, complicated data sets, such as representations of biomolecules. Its foundation is algebraic topology, which maintains the properties of space during continuous transformations. Proposed algebraic topology can extract topological characteristics from molecular structures, notably persistent homology. Machine learning models perform better when constant homology features predict binding affinity and identify active chemicals in virtual screening. Deep convolutional neural networks indicate the properties of molecules, and a unique representation of biomolecules based on their underlying topology is proposed.
A persistent diagram often represents the complex structure, which predicts the protein-ligand binding affinities. Persistent homology was suggested to be employed in a study to extract information from these intricate geometries, which were then used to train a machine learning algorithm to predict the binding affinity of brand-new protein-ligand complexes. This method, known as PerSpect ML, performs better than the alternatives on benchmark datasets. Based on Forman’s Ricci curvature, Wee et al. devised a technique that combines persistent homology with machine learning to extract topological information from intricate geometries. Drug development can be done effectively using this method. In another work, binding affinity was predicted using hypergraph, persistent homology, and machine learning, with the suggested “PSH-ML” outperforming existing approaches on benchmark datasets.
Neural network graph
Graphs can visualize biomedical data, such as protein-protein interactions, drug-disease interactions, drug repurposing, and protein-drug interactions. By displaying complicated links between medications, side effects, drug repurposing, and associated outcomes, knowledge graphs can aid in the creation of new hypotheses. Graphs may easily be translated to chemical structures and are permutation invariant, making them appropriate for representing chemicals and medications.
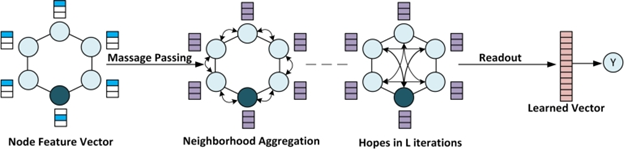
Machine learning algorithms called Graph Neural Networks (GNNs) can be used to find new drugs. GNNs capture a structured representation of atomistic data by encoding pairwise connections rather than locations in a non-Euclidean space. A typical GNN comprises one or more layers that use recursive message passing to learn a permutation-invariant aggregate of nodes from node feature vectors and across neighbors, resulting in a readout operation. Combining atom-related characteristics, including mass, electron count, and charge, can create feature vectors. The spatial structure and feature vectors can be utilized to learn a meaningful representation that can be used to predict a particular property of a molecule or medicine. Molecular characteristics can be predicted using the learned embedding.
GNNs have been extensively exploited in drug discovery applications, including in vivo validation and repurposing medicines as antibiotics. ConvGNNs, MolCLR, and AlphaFold2 are a few examples. The industry standard for handling time series or sequential data, attention-based graph convolution neural networks (GAT), enable networks to take variable-sized inputs by concentrating on the most critical information. Drug binding sites in protein-drug complexes, highly communicative atoms in extensive protein systems, and atoms implicated in binding affinity predictions can all be found using GAT processes.
Modeling a protein-drug complex’s binding pocket
Scientists use the free energy of binding or the energy produced by creating bonds and interactions between proteins and ligands to calculate binding affinities and forecast therapeutic reactions. This energy is calculated by the MD trajectory and MMGBSA tool in Amber, and each residue helps to infer the binding mechanism of a ligand and protein. Geometrical parameters can assess drug efficacy, including drug critical site position, number of interacting atoms, and atom shape. Drug binding sites on a protein are frequently cavities or pockets with concave shapes with more excellent potential interaction surfaces. The Delaunay triangulation method and the Alpha shape can be used to model the complex’s geometry. With contemporary geometrical deep learning, a framework for structure-based docking and drug response analysis can be applied. Modern geometrical deep learning techniques can be used to understand the geometry of protein-drug complexes, providing a framework for structure-based docking and drug response analysis. IC50 values are a frequently used parameter in drug or drug-dose response curves, which depict how an organism or system responds over time to drug exposure.
How to proceed
High-quality annotated labeled datasets and learning representations are necessary for AI in drug discovery, and they can be created through cross-disciplinary collaboration. Clinics, target validation, patient recruiting, and clinical trial design can all be improved with the help of cutting-edge deep learning models, such as those trained on ImageNet. Understanding the disease’s causative chain can help with disease manipulation and reversal, enabling causal machine learning and treatment choices.
For the healthcare sector to properly utilize data science, there is a need for research, collaboration, and professional training in medical and computer science. The long-term goal calls for more workshops on computational biology at the leading AI conferences and new degree programs for AI in drug discovery.
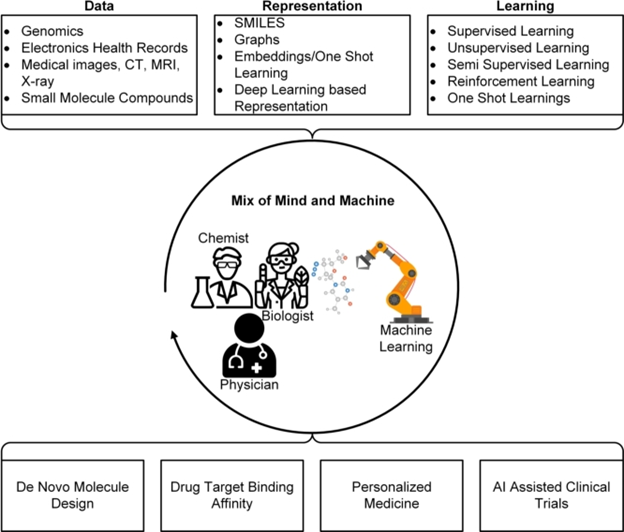
In the healthcare sector, the application of AI-based techniques is growing, revolutionizing processes including medication creation, clinical decision-making, diagnostics, prevention, and therapeutic recommendations. AI applications can speed up the time-consuming and expensive drug design process, previously considered inferior to experimental high-throughput screening and combinatorial chemistry. Some pharmaceutical companies have already used AI; by 2022, revenue from AI-based solutions is expected to total $2.199 billion. Deep neural networks (DNNs) can improve prediction performance when predicting the properties of tiny molecules. At the same time, one-shot learning can be employed without a considerable amount of experimental data. To categorize data and build predictive models, AI is currently being used to produce representations of trials. The future is predicted to see a rise in machine learning, and intense learning, as both the human mind and the computer must cooperate. AI will speed medication development, be a valuable tool in understanding human biology, and act as a catalyst in the fight against disease. However, creating an AI system that can handle multi-objective optimization in a multi-dimensional complicated environment is a significant problem requiring cooperation from numerous academic and industrial disciplines.
Share This Post
Subscribe To Our Newsletter
Get updates and learn from the best
More To Explore
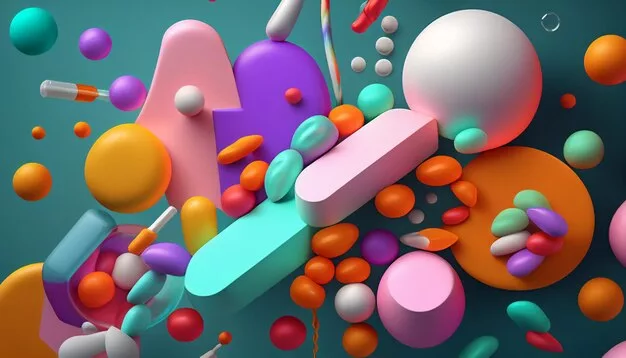
AI improves drug development with symptoms scoring
Free Whitepaper Download AI improves drug development with symptoms scoring Understand the Impact See how AI enhanceing drug development with symptom scoring Personalized Scoring Personalized
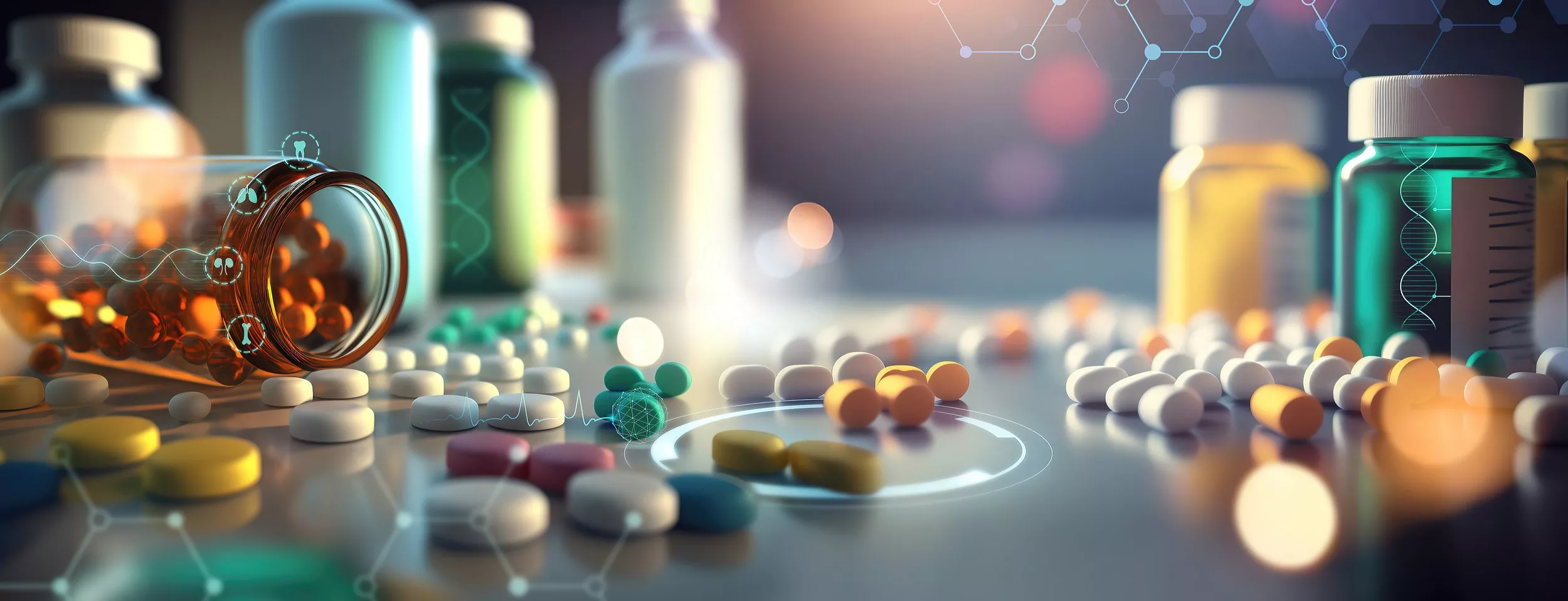
How can AI improve drug optimization with symptom scoring
How can AI improve drug optimization with symptom scoring Intro Increasing medication optimization using symptom rating presents several modern difficulties for the pharmaceutical and healthcare
